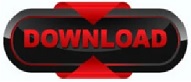
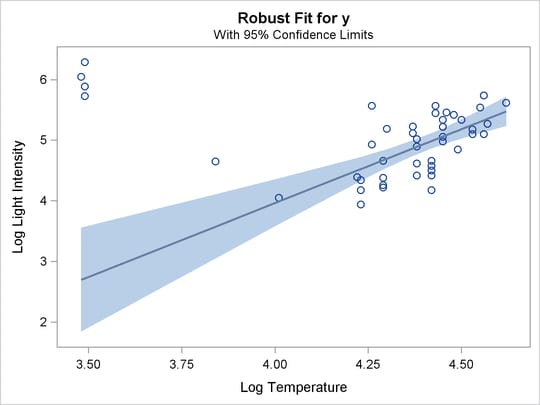
Basement_Area is a significant predictor of SalePrice even after controlling for Lot_Area. The reason is that in the two-predictor model, the parameter estimate for each predictor variable is adjusted for the presence of the other variable in the model. With Basement_Area added to the model, the Lot_Area estimate is notably different than it was in the simple linear regression model (2.87 in the simple regression model and 0.80 in this model), and its p-value no longer shows statistical significance. Our earlier analysis showed that the correlation between Lot_Area and SalePrice was statistically significant. Let's look at the Parameter Estimates tables.
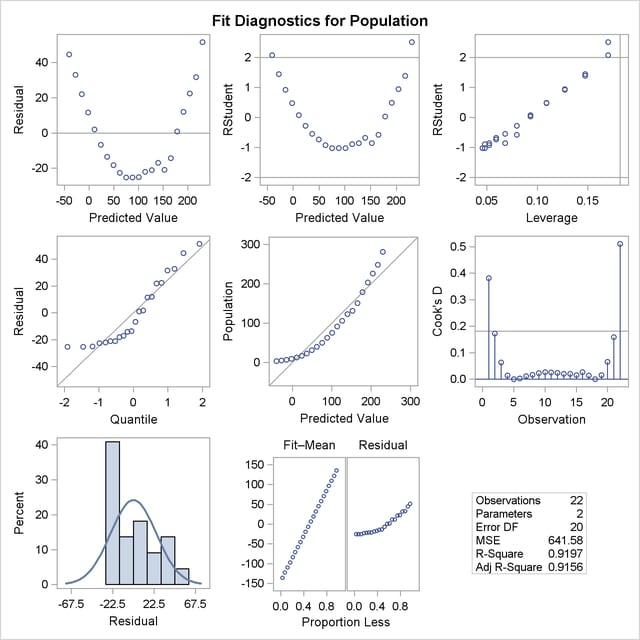
The higher adjusted R-square indicates that adding Basement_Area improved the model enough to warrant the additional model complexity. The adjusted R-square for the multiple regression is higher, at 0.4767.
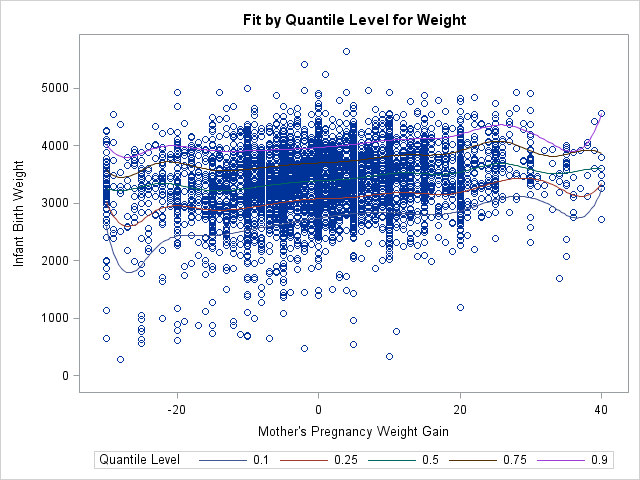
The simpler model with only Lot_Area had an adjusted R-square of 0.061. Is the R-square higher because the new model is better, or simply because the model has more predictors? To find out, compare the adjusted R-square values. Recall from a previous model that Lot_Area alone explained only 6.42%. The R-square of 0.4802, indicates that 48% of the variability in SalePrice can be explained by both Basement_Area and Lot_Area. The Analysis of Variance table shows that this model is statistically significant at the 0.05 alpha level. In the PROC REG step, the MODEL statement specifies SalePrice as the response variable, and Basement_Area and Lot_Area as predictors. We'll save the results of our analyses in an item store, and then use PROC PLM to perform additional analysis. Then we'll use PROC GLM to fit the same model again to show a few additional plots that are not available in PROC REG. In this program, we'll use PROC REG to run a linear regression model with two predictor variables.
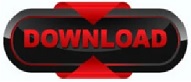